Gen AI’s memory wall – DataScienceCentral.com

Some takeways after MEMCON 2024
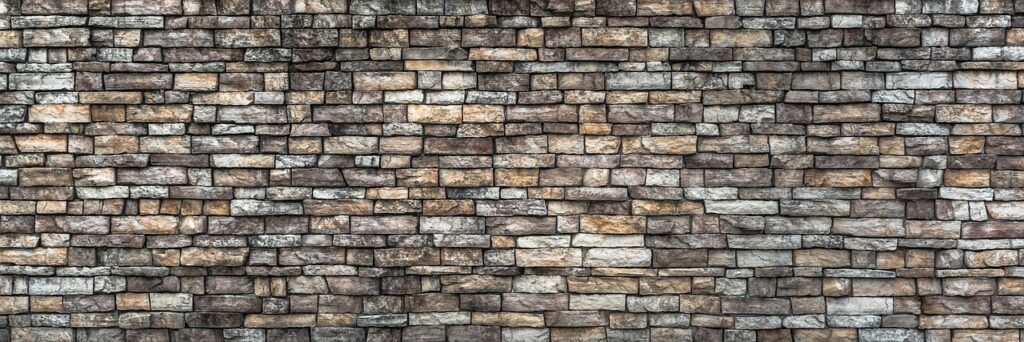
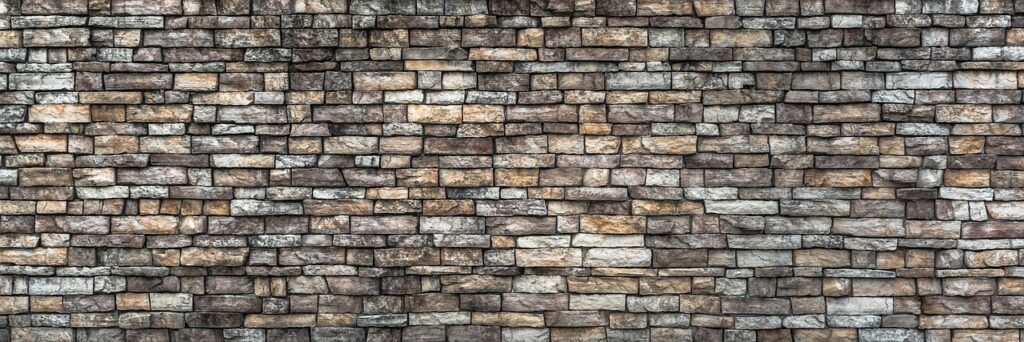
Image by jeonsango on Pixabay
All through an interview by Brian Calvert for a March 2024 piece in Voxnative climate lead and AI researcher at Hugging Face Sasha Luccioni drew a stark comparability: “From my very personal evaluation, what I’ve found is that switching from a non-generative, good outdated type quote-unquote AI technique to a generative one can use 30 to 40 situations additional energy for the exact same course of.”
Calvert components out that gen AI involving large language model ( LLM) teaching requires a whole lot of iterations. Furthermore, lots of the knowledge in proper this second’s teaching models is form of duplicated. If that data have been completely contextualized and thereby deduplicated, akin to via a semantically fixed knowledge graph, as an example, far smaller teaching models and training time could possibly be ample.
(For additional data on such a hybrid, neurosymbolic AI technique may assist, see “How hybrid AI may assist LLMs grow to be additional dependable,” https://www.datasciencecentral.com/how-hybrid-ai-can-help-llms-become-more-trustworthy/.)
So-called “foundational” model use requires actually foundational enhancements that by definition will in all probability be slower to emerge. For now, LLM clients and infrastructure suppliers are having to make use of costly methods merely to keep up tempo. Why? LLM demand and model dimension is rising so quickly that functionality and bandwidth are every at a premium, and every of those are onerous to return by.
Regardless of the costs, inefficiencies and inadequacies of LLMs, sturdy market demand continues. Hyperscale data services proceed to enhance their facilities as shortly as potential, and the roadmap anticipates additional of the equivalent for the next few years.
Although developments in smaller language fashions are compelling, the bigger is more healthy model dimension sample continues. All through his keynote at Kisaco Evaluation’s MEMCON 2024 event in Mountain View, CA in March 2024, Ziad Kahn, GM, Cloud AI and superior Strategies at Microsoft, well-known that LLM dimension grew 750x over a two-year interval ending in 2023, in distinction with memory bandwidth progress of merely 1.6x and interconnect bandwidth of 1.4x over the equivalent interval.
The interval of trillion-feature LLMs and GPU superchip giant iron
What the model dimension progress situation implies is LLMs launched in 2023 which could be over a trillion choices each.
Curiously, the MEMCON event, now in its second 12 months, had many high-performance computing (HPC) audio system who’ve normally been centered for years on giant scientific workloads at giant labs similar to the US Federally Funded Evaluation and Development Services (FFRDCs) akin to Argonne Nationwide Laboratory, Lawrence Berkeley Nationwide Laboratory, and Los Alamos Nationwide Laboratory. I’m not used to seeing HPC audio system at events with mainstream attendees. Apparently that’s the on the market cadre that may stage the easiest way forward for now?
FFRDC funding reached $26.5 billion in 2022, in line with the Nationwide Science Foundation’s Nationwide Center for Science and Engineering Statistics. A couple of of the scientific data from these FFRDC’s is certainly now getting used to educate the model new trillion-feature LLMs.
What’s being constructed to cope with the teaching of these giant language fashions? Racks like Nvidia’s liquid-cooled GB200 NVL 72, which includes 72 Blackwell GPUs, 36 Grace Hopper CPUs, and an whole full of 208 billion transistors, which can be interconnected with the help of fifth-generation, bi-directional NVLink. Nvidia launched the model new rack system in February 2024. CEO Jensen Huang often called the NVL 72 “one giant GPU”.
This mannequin of LLM giant iron, as giant and intimidating as a result of it appears, actually attracts pretty a bit a lot much less power than the earlier period. Whereas a 1.8T LLM in 2023 may want required 8,000 GPUs drawing 15 megawatts, proper this second a comparable LLM can do the job with 2,000 Blackwell GPUs drawing 4 megawatts. Each rack incorporates nearly two miles of cabling, in line with Sean Hollister, writing for The Verge in March.
My impression is that lots of the innovation on this rack stuffed full of processor + memory superchips consists of considerable packaging and interconnect design innovation, along with home, specific provides and extra cabling the place they’re essential to deal with thermal and signal leakage issues. Further primary semiconductor memory experience enhancements are going to take various years to kick in. Why? Numerous thorny factors must be addressed on the same time, requiring design points that haven’t really been labored out however.
Current realities and future objectives
Simone Bertolazzi, Principal Analyst, Memory at chip commerce market evaluation company Yole Group, moderated an illuminating panel session near the highest of MEMCON 2024. To introduce the session and provide some context, Bertolazzi highlighted the near-term promise of high-bandwidth memory (HBM), a longtime experience that provides higher bandwidth and reduce power consumption than completely different utilized sciences on the market to hyperscalers.
Bertolzazzi anticipated HBM DRAM in unit phrases to develop at 151 % 12 months over 12 months, with earnings rising at 162 % via 2025. DRAM usually as of 2023 made up 54 % of the memory market in earnings phrases, or $52.1 billion, in line with Yole Group. HBM has accounted for about half of full memory earnings. Complete memory earnings might attain nearly $150 billion in 2024.
One in every of many particulars panelist Ramin Farjadrad, Co-Founder & CEO at chiplet construction innovator Eliyan made was that processing tempo has elevated 30,000x over the past 20 years, nonetheless that DRAM bandwidth and interconnect bandwidth have solely elevated 30x each all through that exact same time interval. That’s the manifestation of what many on the conference often called a memory or I/O wall, an absence of memory effectivity scaling merely when these 1T learning fashions demand it.
This is not to say that there are a collection of long-hyped memory enhancements which could be sitting on the sidelines on account of the enhancements are solely confirmed in narrowly outlined workload conditions.
The most effective state of affairs is that utterly differing types of memory might very properly be built-in proper right into a single heterogeneous, multi-purpose memory materials, making it potential to match utterly completely different capabilities to utterly completely different needs on demand. That’s the dream.
Not surprisingly, the very fact seems to be that memory utilized in hyperscale data coronary heart capabilities will nonetheless be a longtime tech hodgepodge for awhile. Mike Ignatowski, Senior Fellow at AMD, did seem hopeful about getting earlier the 2.5D bottleneck and into 3D packaging, along with photonic interconnects and co-packaged optics. He recognized that HBM acquired started in 2013 as a collaboration between AMD and SK Hynix.
The talked about completely different to HBM, Compute Categorical Hyperlink (CXL), does present the abstraction layer essential to a extremely heterogeneous memory materials, nonetheless it’s early days however, and it seems the effectivity CXL gives doesn’t however look at.
DRAM chief Samsung, with nearly 46 % of the market inside the closing quarter of 2023, in line with The Korea Monetary Day-to-day, is outwardly planning to increase HBM wafer begins by 6x by subsequent 12 months. Doesn’t seem seemingly that they’ll be closing the demand gap any time shortly.