Defining transformation in the era of AI + FAIR data

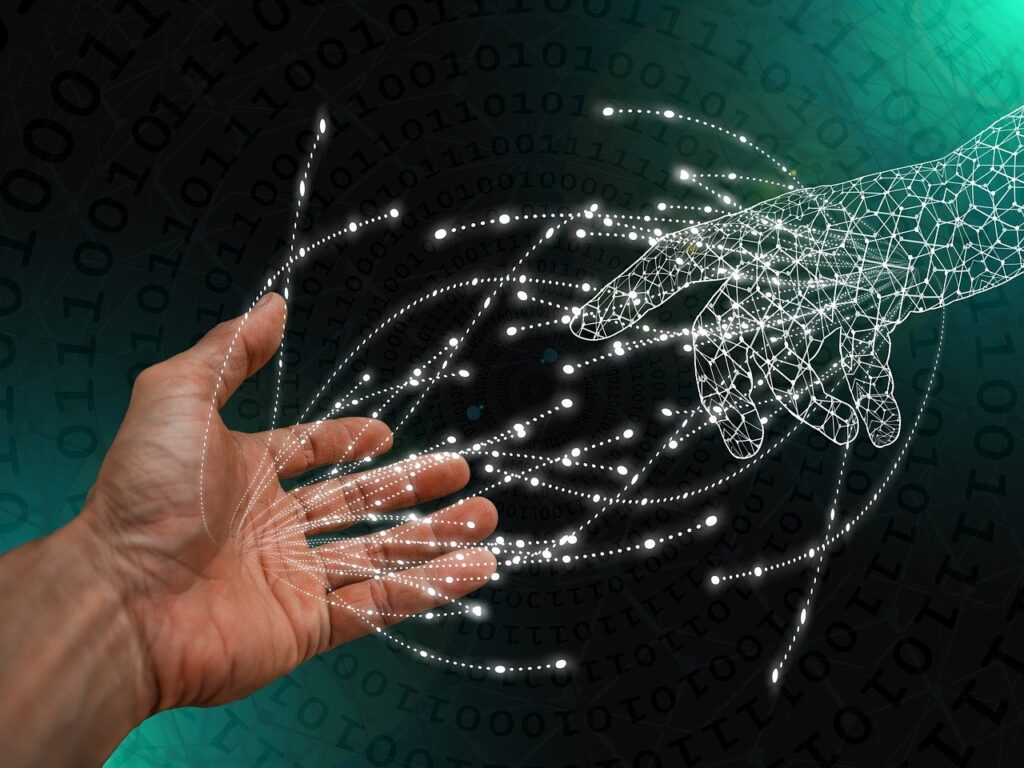
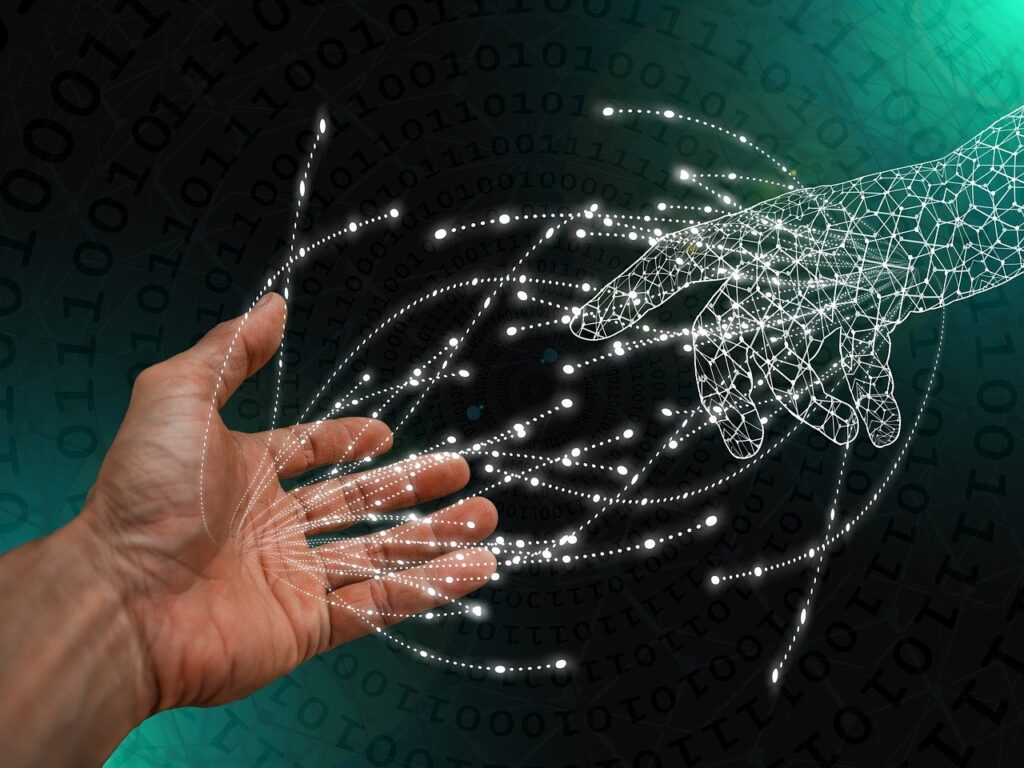
Image by Gerd Altmann from Pixabay
Every week, I watch rising tech markets evolve and try and ponder what the changes suggest by the use of precise enterprise impression on large enterprises and different types of corporations as correctly. Being a market watcher has been a conduct of mine for almost 25 years now as a longtime forecaster, researcher and tendencies analyst.
It’s biggest as a market analyst to hint how packages as a whole evolve, fairly than merely explicit particular person utilized sciences. Doesn’t make sense to undertake the half with out consideration to its impression on your entire.
The capabilities of a given AI system in that sense, I’ve found, hinge on ample portions of full, associated data that’s findable, accessible, interoperable and reusable (FAIR). For those who occur to don’t have the correct of FAIR data to make the AI system work the easiest way it should, you don’t have an advanced AI system, interval.
Furthermore, companies who’ve contributed most to FAIR data initiatives have devoted to necessities and an information graph technique.
Apparently, Gartner in its 2023 Hype Cycle for Artificial Intelligence plotted information graphs near the tip of the Trough of Disillusionment, implying a stage of maturity most newer AI-related utilized sciences don’t have.
In its Affect Radar for 2024, Gartner moreover put information graphs inside the bullseye with generative AI, the two utilized sciences Gartner decided promised the most important impression potential this yr. The implication I’m taking from these Affect Radar + Hype Cycle findings is that information graphs must be the following priority than generative AI. Finally, generative AI has merely entered the Trough of Disillusionment, and gen AI needs prime quality, contextualized data merely as loads as another sort of AI does.
Seven tenets of transformation inside the AI interval
If AI is set by data, what’s digital transformation with out data layer transformation?
For the earlier 15 years, enterprise data layer transformation has been a key focus of mine, notably transformations that comprise the utilization of semantic graph databases or information graphs further normally. I’ve had that focus because of data maturity writ large is a precondition to enterprise intelligence, analytics and AI success.
As I’ll describe shortly, using a fingers on information graph technique can change an enterprise’s complete data administration mentality. Committing to such an technique makes packages designed to harness the ability of AI as a whole further scalable and manageable
These seven tenets of enterprise transformation inside the AI interval underscore every the situation we’re really in and what it ought to take to make substantive AI progress:
- Whatever the hype since 2019, we nonetheless have solely slender artificial intelligence, and nonetheless solely pure language processing, not understanding.
- To have the flexibility to make biggest use of the AI that exists requires that each enterprise assemble a recent information foundation, i.e., data that’s logically associated, contextualized and extensible with the help of shared, interlinked, symbiotic graph data fashions.
- Organizations can’t be data mature within the occasion that they’re not in data sharing mode at any time when they’re typically, along with pushing the data sharing envelope. Holding onto data doesn’t make sense in case you’re not sharing with the people who need what that data can current.
- Transformation doesn’t matter in case you’re not providing enterprise-wide and even boundary-crossing transparency inside the course of.
- It doesn’t make sense to buy “AI” with out having the ability first to feed the AI among the best, top of the range, associated, disambiguated data you’ll.
- A number of the AI software program program suppliers available on the market, sadly, aren’t centered on serving to you resolve your data points.
- Most enterprises have massive, unresolved data points. Subsequently, most enterprises acquired’t revenue all that loads from AI.
Occasion case analysis from enterprises devoted to multi-year, AI-era transformation
Enterprises who’ve demonstrated years of dedication to information graph-based data contextualization, administration and sharing are amongst these organizations most worth emulating. Underneath are two compelling newest examples.
Aerial drone interdiction information sharing on the US Division of Homeland Security (DHS). DHS on the Semantic Arts’ 2023 Information-Centric Construction Dialogue board shared particulars of its efforts to mix heterogeneous, real-time intelligence sources at scale using a standards-based information graph technique. DHS was centered on detecting smuggling drones crossing the US-Mexican and US-Canadian borders and shortly alerting brokers to the landing web sites so the smugglers might very nicely be apprehended.
Port-wide emissions monitoring using information graph-based digital twins and brokers. Portsmouth Ports now shows and shares nicely timed metrics on emissions updates to a broad differ of enterprise clients. Armed with this information, transport companies, as an illustration, can resolve which vessels, when and the place, are out of compliance with emissions administration requirements. This stage of temporal + spatial ingredient is essential to hold assorted transportation varieties and the ports themselves into compliance with UK guidelines. (See the IOTICS Portsmouth Ports use case at https://www.datasciencecentral.com/preconditions-for-decoupled-and-decentralized-data-centric-systems for further information.)
Further case analysis like these could also be found on my Information Science Central internet web page at https://www.datasciencecentral.com/author/alanmorrison/ Let me know your concepts. If these case analysis help, or if there are totally different case analysis alongside these equivalent traces you’d want to stage out, please let me know.